There is an excessive selection of information offered to telecom operators on the status, efficiency and health of their networks; at different speeds and levels of granularity; concentrated on end-user-experience, peak network ability, or lots of other prospective signs.
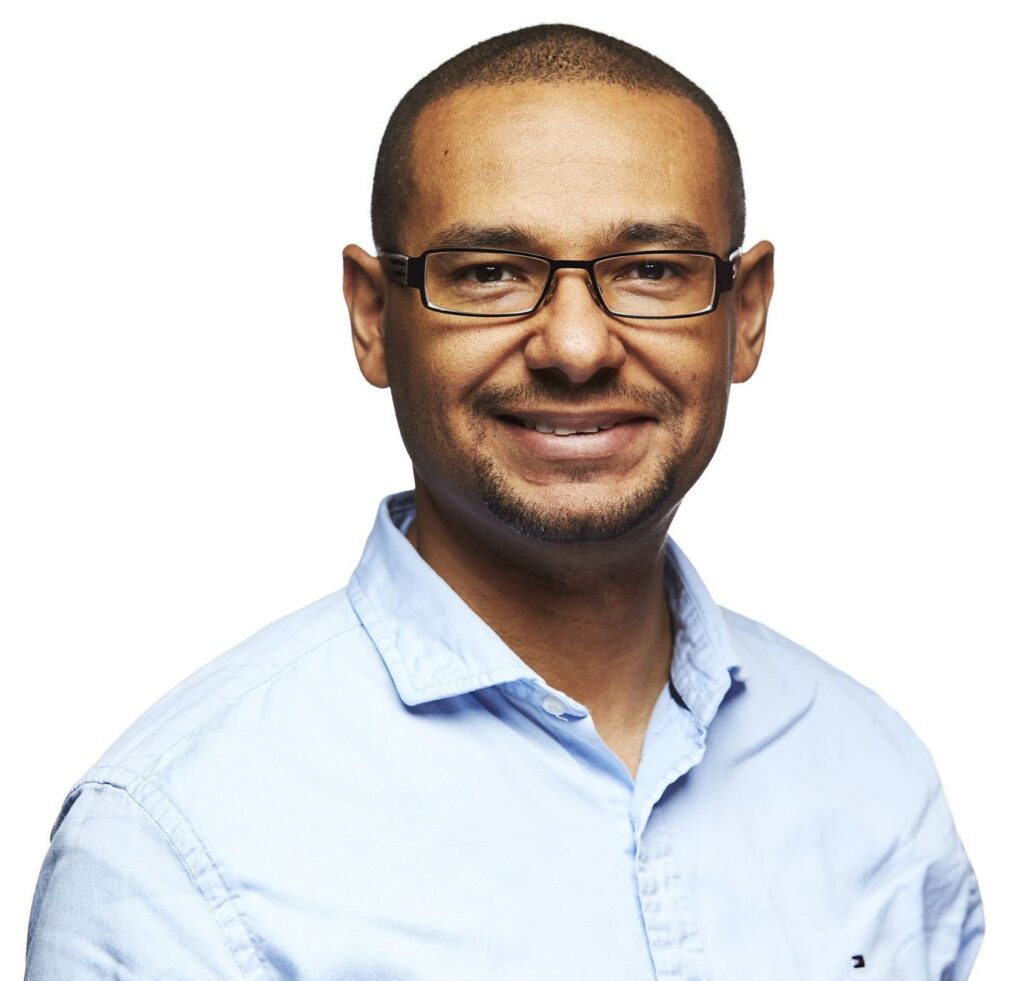
Operators face significant difficulties in making the very best usage of that information and equating it into actionable intelligence to verify, guarantee and enhance network operations. RCR Wireless News connected to Ayodele Damola, director of synthetic intelligence/machine knowing technique at Ericsson, for his point of view on those difficulties and the existing landscape and patterns in event, evaluating and leveraging network information in addition to using AI and ML in telecom networks.
This Q&A was performed through e-mail and has actually been gently modified.
RCR: As the market moves even more into 5G and 5G Standalone releases, in addition to disaggregation and cloud-native networks, how would you explain the difficulties surrounding browsing network-related information? Is it a matter of brand-new sources (i.e., microservices causing more information granularity), brand-new volume or scale of information, or the velocity/speed at which information is offered– or some other aspect?
The primary difficulty browsing network information is the boost in intricacy. As 5G gains traction around the world, interaction provider (CSP) networks are ending up being a lot more complicated. The intricacy is because of the brand-new set of services being provided, the boost in number and kinds of gadgets in the network, schedule of brand-new spectrum frequencies and bands, and the development of physical networks to virtualized networks. This intricacy relates to a boost in the volume of network information produced; network information produced in various amount of time in addition to the existence of more range of network information. If we take, for instance, the recommendation specification count development in 3GPP radio gain access to networks (RAN)– we saw that with 2G networks when we had simply a couple of hundred recommendation criteria that required to be set up. In 5G, that number has actually grown to numerous thousand criteria. In 6G, we expect an even bigger variety of recommendation criteria. Boost in intricacy is likewise shown in ‘oversupply of information’– it ends up being challenging to discover appropriate information without ingenious filtering and aggregation. Another concern is the absence of standardization of information throughout exclusive supplier devices causing troubles in leveraging the information for insights. Yet another concern is information management– today, it is an afterthought including fragile system details (SI) driven pipelines which mishandle. Generally, information is duplicated and difficult to govern. All these elements put brand-new needs on the level of effort required to handle and manage the network, and consequently result in an increased CSP operation cost (OpEx) and ultimately increased capital expenditure (CapEx). It is ending up being clear that it will no longer be possible to handle networks in a tradition method where network engineers take a look at control panels and make modifications by hand to the network. This is where an innovation like AI goes into the image, an innovation that allows automation and consequently lowers intricacy.
RCR: Do you believe that telecom operators, by and big, have an excellent deal with on their network information? What do you believe they succeed, and where exists space for enhancement?
Access to network information is a crucial part of many CSP techniques today. Network information from multi-vendor networks has actually been rather of an obstacle for CSPs due to the fact that each supplier has somewhat various network node user interfaces– for this reason, the format and syntax of the information might be various throughout various suppliers. Typically, CSPs do not have a constant technique for information determination and direct exposure. Various storage systems (e.g., information lakes) and retention policies make complex the scalable handling of information volumes. In the future, open requirements assure to relieve this difficulty, particularly by the intro of the Service Management and Orchestration (SMO) structure specified by the ORAN Alliance, which supplies a set of distinct user interfaces allowing CSPs to gain access to and act upon information from both purpose-built and virtualized multi-vendor and multi-technology networks. Network information is exposed through open user interfaces (e.g., R1, A1, O1, O2 etc.) to the various SMO functions.
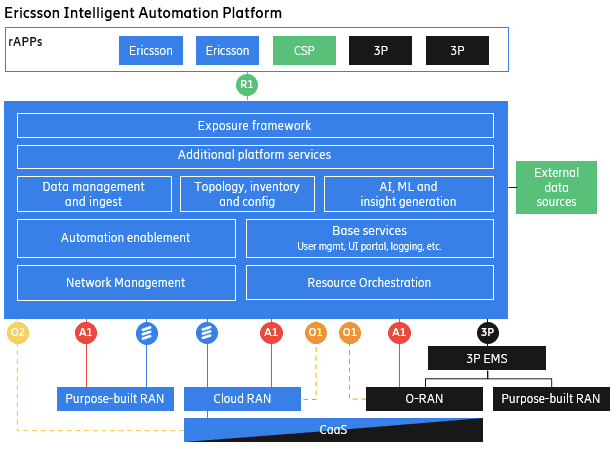
Within the SMO (our execution is called the Ericsson Intelligent Automation Platform) the Information management and consume function allows CSPs to effectively and firmly consume and handle information. The AI/ML and insight generation function allows the capability to procedure and evaluate the information, obtaining insights and helping with actuations.
RCR: What do network operators wish to utilize their information for? Can you offer the leading 3 usages for network-related information that are the main interest of MNOs?
Marketing research previously commissioned by Ericsson reveals that CSPs utilize network information throughout lots of utilize cases, with the leading 3 being:
- Client Experience Management: Service assists CSPs anticipate consumer fulfillment, find experience concerns, comprehend source, and instantly takes the next finest action to enhance experience and functional performance causing churn decrease and increased consumer adoption.
- Security/Fraud & & Earnings Guarantee: Addresses security management and earnings guarantee consisting of billing and charging.
- Cloud & & IT Operations: Automation of cloud and IT management operations consisting of administrative procedures with assistance for software and hardware, and the fast seclusion of faults.
In addition, other essential usage cases consist of Network Management & & Operations, Business Operations, Service Guarantee for RAN & & Core, Network Style and Optimization, RAN Spectrum and Traffic Management.
RCR: What function is AI/ML playing in networks at this minute in time? Individuals are extremely thinking about the capacity– what is the existing truth of useful AI/ML usage in telecom networks, and could you offer some real-world examples?
AI/ML assures substantial capacity when it pertains to enhancing and automating CSP networks. The market is still in its early days, however some issues that have actually been resolved with AI/ML reveal significant gains. The complete systemization of CSP networks based upon AI/ML, likewise called AI-native networks, is still some years away. When it pertains to the RAN particularly, Ericsson thinks that AI/ML will play a crucial function in the following locations:
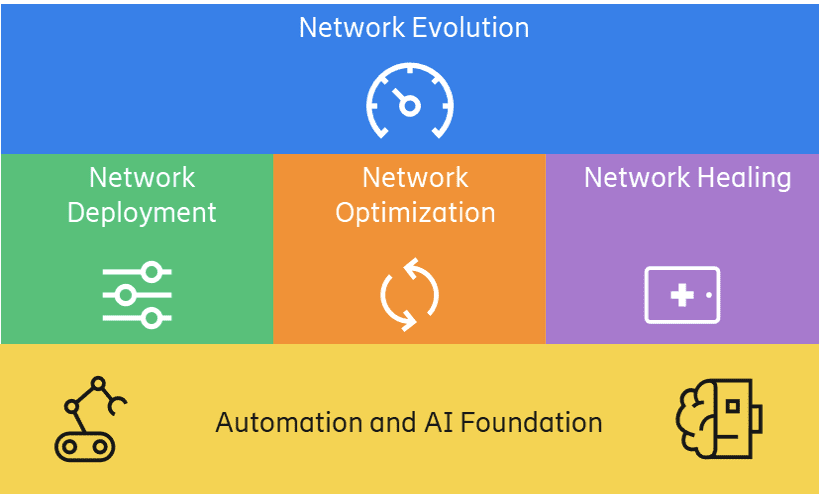
- Network development: Improves network preparation with more effective RF preparation, website choice and capability management. Enhances network and service efficiency and allows brand-new earnings by information driven and intent-based insights and suggestions.
- Network implementation: Deals with provisioning and life process management of complicated networks with ideal expenses and speed to market.
- Network optimization: Smart self-governing functions to enhance consumer experience and roi, e.g., RF shaping, traffic and movement management, energy performance and so on
- Network recovery: Service connection and resolution of both standard and complicated occurrences, providing high schedule while keeping the operation expenses at a minimum.
- AI and automation structure: Makes it possible for faster TTM for– and rely on– high efficiency AI and automation utilize cases by ways of openness and versatility.
In addition, Ericsson thinks CSPs will gain from an end-to-end handled services operations service allowing the smart management of CSP networks and services to supply remarkable connection and user experience. Powered by innovative analytics and artificial intelligence algorithms, CSPs will gain from the capability to anticipate prospective network concerns triggered by hardware, software application, or external elements such as weather condition disruptions or consumer habits patterns.
Practical AI/ML utilize in telecom networks today:
- Capability Preparation: Supplies the capability to carry out proactive preparation based upon traffic projection in mix with AI/ML forecast of usage KPIs. The result will be the maximum capability Expense to satisfy a specific QoS level. Projection forecasts, traffic jam recognition and network dimensioning are the primary usage cases. Advantages consist of 20-40% CapEx cost savings less provider growths compared to standard method, and 83% increased functional performance increased functional performance on dimensioning jobs.
- Efficiency Diagnostics: An option that evaluates CSPs’ RAN to find and categorize cell concerns. Recognized concerns are even more examined to source level, allowing quickly and precise optimization of end-user efficiency. Advantages consist of: 30% boost in capability per optimization full-time staff member (FTE), and 15% much better downlink speed in cells with concerns.
- Better spectrum performance: By gathering nearby cell information in genuine time, it is possible to enhance radio link efficiency utilizing pattern acknowledgment. Advantages consist of: 15% enhanced spectrum performance and 50% increased cell edge DL throughput.
- Sustainability: A self-governing system utilizing AI/ML innovations and closed-loop automation to decrease everyday radio network energy usage by approximately 25% with absolutely no effect on user experience.
When it pertains to the capacity of AI/ML in CSP networks, we picture a journey with numerous actions:
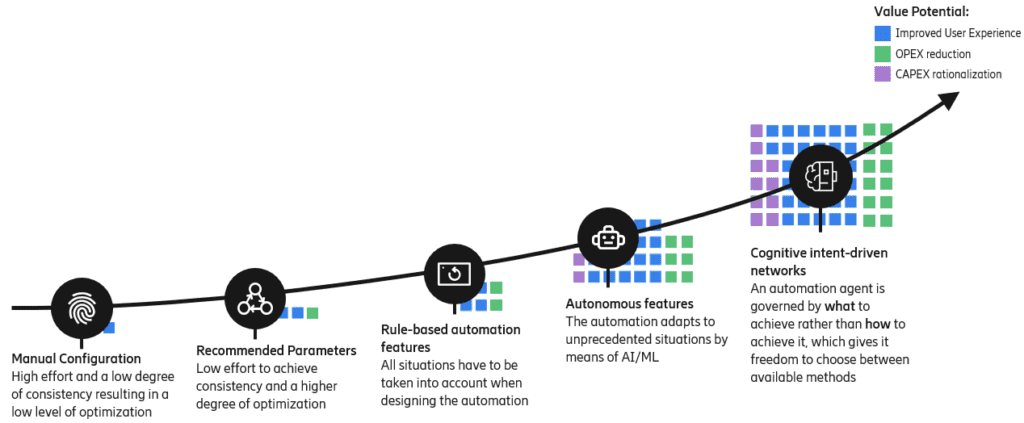
In the earliest action, there was total human intervention as the network was by hand set up. In the next action, the network setup was still carried out by human beings, however the effort level was minimized to the setup of a suggested set of criteria. In the rule-based action, the human function was to produce a set of guidelines which then managed the network; this needed that the human designers have a deep understanding of how the network functions. We are presently transitioning to the action with self-governing functions where we have AI/ML designs adjusting to brand-new circumstances and consequently providing CPS more automatic control. Moving forward, the vision is that the network will develop to totally self-governing without any human intervention besides setting intents by which the network runs– generally, supplying the ‘what’ requirements to the network with the network carrying out the required ‘how’ actuation and control. It is a journey comparable to the development of vehicles from by hand managed to totally self-driving.
RCR: In regards to edge vs. central cloud, is most information processing still centralized or do you see things in fact ending up being more dispersed and occurring at the edge? Is it various for telco work vs. business work?
It is necessary to compare information collection, usually for the function of training an AI/ML design, and reasoning, which involves acting upon brand-new information by an experienced design. The procedure of gathering information will require cleansing and arranging the information and after that utilizing this information to train designs. While the information collection will take place out in the network in a dispersed way, the information processing will usually be done central. After the design is trained, the implementation of the design in the network might be either dispersed or centralized, depending upon the usage case. Offered the big volume of network information and provided its real-time nature, inferencing of telco work will usually be more dispersed compared to business work.
RCR: There was for some years a huge push towards “information lakes” and keeping as much information as possible to sort through for service intelligence. Is this the case for network information too, or exists more choice for real-time intelligence? What does “actual time” in fact suggest today, how close can it get?
The choice genuine time intelligence will depend upon the usage case. Network information is produced throughout the network in various amount of time, and we can categorize usage cases based upon the network information into fast-loop usage cases (split second timeframe) and slow-loop usage cases (timeframe of days or perhaps weeks) and all the in-between. The nature of the usage cases throughout various timeframes will vary considerably. An actual time or fast-loop usage case is, for instance, radio scheduling made in split seconds carried out in your area within a network node and usually totally automated i.e., done without a human in the loop. Slow-loop usage cases will be focused more on long term network patterns like network benchmarking, needing network broad coordination with more time offered to decide and will likely require interaction with a human. Information lakes are then matched for the 2 upper loops in the image listed below, while the time level of sensitivity of both the information and the choices made in the 2 lower loops speak versus determination of the decision-bearing information in any kind of information lake.
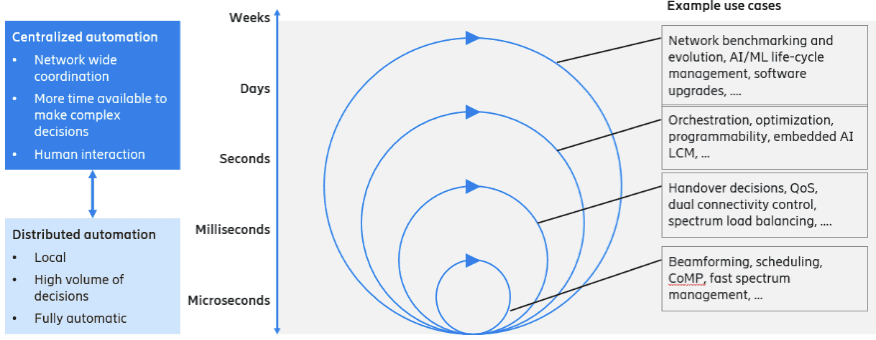
RCR: What information requirements, difficulties or modifications should telecom operators be preparing for now that you see being available in the next 3-5 years?
I see 3 things:
- Quality information: CSPs are challenged with how to specify and establish a quality information method from which all AI options can be provided. The concern is that information is still included in silos throughout many CSPs, from tradition systems to brand-new systems. AI/ML will just make a distinction if tidy information is offered from all sources. For this reason, investing the time in and after that training of AI algorithms with the best information will be necessary to minimizing incorrect alarms and in enhancing AI efficiency. A surrounding issue is the concern of appropriate information– a few of the information produced has extremely little to no entropy (i.e., its effectiveness is restricted) and recognizing such information is a continuous difficulty.
- Information platform: All CSPs need a robust platform to aggregate, sterilize, and evaluate the information. While there are numerous prospective options in the market, there are issues of personal privacy, security, and supplier lock-in that make information platform choice challenging.
- Information technique: Numerous CSPs do not have an end-to-end information technique which covers information governance, simplification and automated collection, and information analysis. While some CSPs have a Chief Data Officer (CDO), lots of CSPs have actually not yet had the ability to impose a companywide information technique throughout different companies causing detached islands of information.